Leveraging Machine Learning for Predictive Quality Control
The role of a Production Planner in this scenario is crucial, as they are tasked with orchestrating the complex interplay of resources, schedules, and processes to ensure efficient production while maintaining stringent quality standards. Traditional quality control methods have their limitations, often reactive and prone to human error. However, with advancements in technology, particularly the integration of machine learning algorithms, a paradigm shift towards predictive quality control is within reach.
This blog looks into the integration of machine learning algorithms with PlanetTogether, a production planning software, and major ERP, SCM, and MES systems like SAP, Oracle, Microsoft, Kinaxis, and Aveva, to enhance quality control in chemical manufacturing.
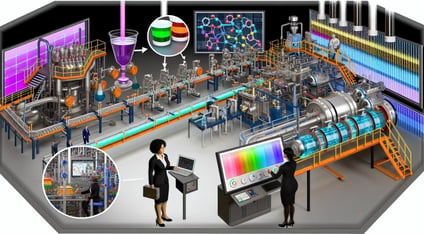
Predictive Quality Control
Predictive quality control entails leveraging historical data, real-time monitoring, and advanced analytics to foresee potential quality deviations and take proactive measures to mitigate them. Unlike traditional quality control methods, which rely on post-production inspection and correction, predictive quality control empowers manufacturers to anticipate defects, identify root causes, and optimize processes in real-time. This proactive approach not only reduces waste and rework but also enhances overall product quality and customer satisfaction.
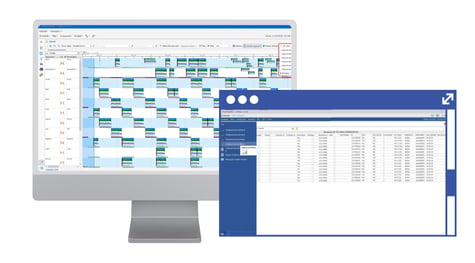
Integration of Machine Learning with PlanetTogether and ERP/SCM/MES Systems
The integration of machine learning algorithms with production planning software like PlanetTogether and ERP, SCM, and MES systems opens up a realm of possibilities for predictive quality control in chemical manufacturing. Here's how:
Data Integration and Aggregation
Machine learning algorithms thrive on data. By integrating PlanetTogether with ERP, SCM, and MES systems, manufacturers can aggregate vast amounts of data from various sources, including production schedules, inventory levels, quality metrics, and external factors like market demand and raw material availability.
SAP, Oracle, Microsoft, Kinaxis, Aveva, and other major ERP, SCM, and MES systems serve as repositories of valuable operational data, which, when combined with production planning data from PlanetTogether, provide a comprehensive view of the manufacturing ecosystem.
Predictive Modeling and Analysis
Machine learning algorithms can analyze historical data to identify patterns, correlations, and anomalies associated with quality deviations.
By training predictive models on integrated datasets, manufacturers can forecast potential quality issues, such as equipment failures, process variations, or raw material inconsistencies.
These models can also factor in external variables, such as weather conditions or supplier performance, to enhance prediction accuracy.
Real-time Monitoring and Alerts
Integrating machine learning algorithms with real-time monitoring systems enables continuous assessment of production processes and quality parameters.
As data streams in from sensors, IoT devices, and production equipment, machine learning models can analyze this information in real-time to detect deviations from expected quality standards.
Instantaneous alerts can be generated within PlanetTogether or ERP/SCM/MES interfaces to notify Production Planners and operators of potential quality issues, enabling timely intervention.
Root Cause Analysis and Optimization
Beyond predicting quality deviations, machine learning algorithms facilitate root cause analysis by correlating process variables with quality outcomes.
By identifying underlying factors contributing to quality issues, manufacturers can implement targeted corrective actions to address root causes effectively.
Moreover, continuous feedback loops between predictive models and production systems enable ongoing process optimization, driving continuous improvement in quality and efficiency.
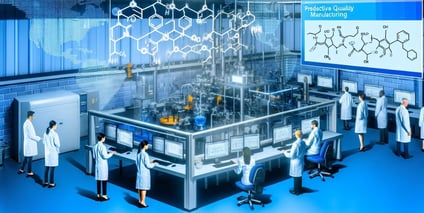
Benefits by Leveraging Predictive Quality Control
Reduction in Waste and Rework: Proactive identification of quality deviations minimizes production losses and improves resource utilization.
Enhanced Product Quality: Predictive models enable early detection of quality issues, ensuring that only high-quality products reach the market.
Operational Efficiency: Optimized production processes and timely interventions streamline operations, leading to cost savings and improved competitiveness.
Data-driven Decision-making: Insights derived from machine learning algorithms empower Production Planners and decision-makers to make informed choices and drive continuous improvement initiatives.
The integration of machine learning algorithms with production planning software like PlanetTogether and major ERP, SCM, and MES systems heralds a new era of predictive quality control in chemical manufacturing. By harnessing the power of data analytics, real-time monitoring, and advanced algorithms, manufacturers can proactively anticipate quality deviations, optimize production processes, and enhance product quality.
As Production Planners navigate the complexities of modern manufacturing, embracing predictive quality control offers a strategic advantage in achieving operational excellence and sustaining competitive advantage in the dynamic chemical industry landscape.
Are you ready to take your manufacturing operations to the next level? Contact us today to learn more about how PlanetTogether can help you achieve your goals and drive success in your industry.
LEAVE A COMMENT